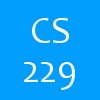
Welcome! This is your class description

Go back to:CS229: Machine Learning
Topics: statistical pattern recognition, linear and non-linear regression, non-parametric methods, exponential family, GLMs, support vector machines, kernel methods, model/feature selection, learning theory, VC dimension, clustering, density estimation, EM, dimensionality reduction, ICA, PCA, reinforcement learning and adaptive control, Markov decision processes, approximate dynamic programming, and policy search. Prerequisites: linear algebra, and basic probability and statistics.
Go back to: CS229: Machine Learning
Join thousands of students profiting from perkmylife resources
Courses, notes, Q&A groups - all you need to study efficiently and achieve the goals!
- Explore our biggest groups
- and much more...